You may not realize it, but the next few images you encounter could be the work of artificial intelligence. Recent estimates suggest a staggering 34 million images are generated daily using AI. Since 2022, photo-uploading services have increasingly grappled with misusing these AI-created visuals. This trend underscores the pressing need for dependable methods to identify synthetic images. Let’s delve into four practical solutions currently in use or under consideration for implementation.
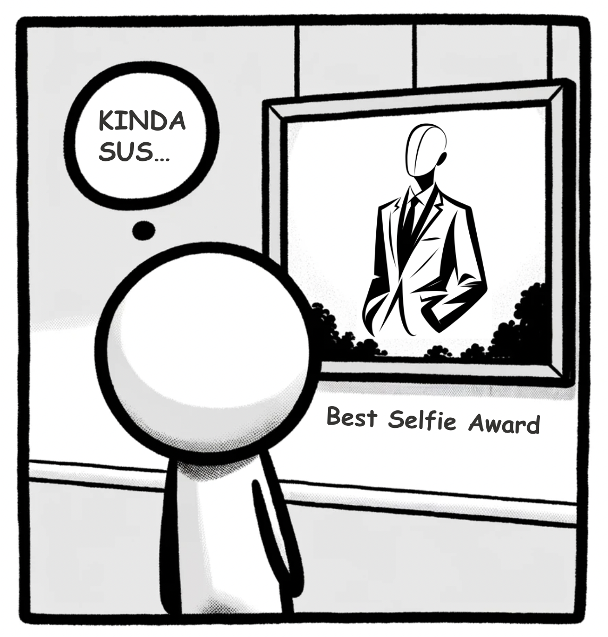
1. User-Declared AI Image Tagging
- Explanation: This method relies on the honesty of users, allowing them to tag their uploads as AI-generated. It's a straightforward approach and has been in practice by platforms like Pixiv for years.
- Example: Upon uploading an artwork, Pixiv users can tag it as “AI-generated,” helping in content categorization.
- Con: The obvious downside is the reliance on users' honesty. Nothing can stop someone from uploading an AI-generated image without the proper tag. In practice, service providers will often want to implement additional detecting methods on their behalf.
2. EXIF Data Analysis
- Explanation: Every digital photo contains EXIF data which provides information about the camera used, settings, and more. Analyzing this data can sometimes help distinguish real images from AI-generated ones.
- Example: A photo with complete and consistent EXIF data indicating it was taken with a known camera model might be considered authentic.
- Con: Creators of AI images can still fake EXIF data, rendering this method less effective against determined attempts to deceive. Uploading the EXIF also provides sensitive data such as your location information, which some users may not like.
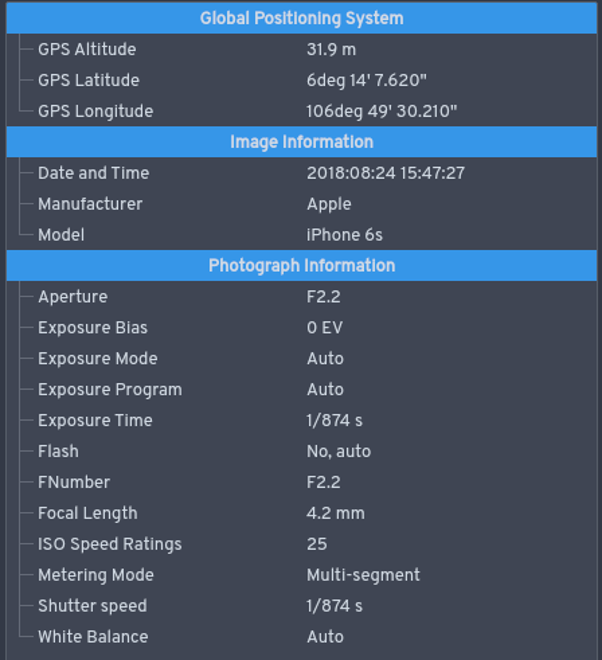
3. Use AI to Fight AI
- Explanation: Using advanced AI models to detect signs of AI generation in images. Services like AI or Not analyze images to spot telltale signs of AI involvement.
- Example: When a user uploads an image, the system scans it and provides a likelihood score of being AI-generated based on various AI model markers.
- Con: While effective, this method can be costly and is not foolproof. There's a chance of sophisticated AI-generated images slipping through.
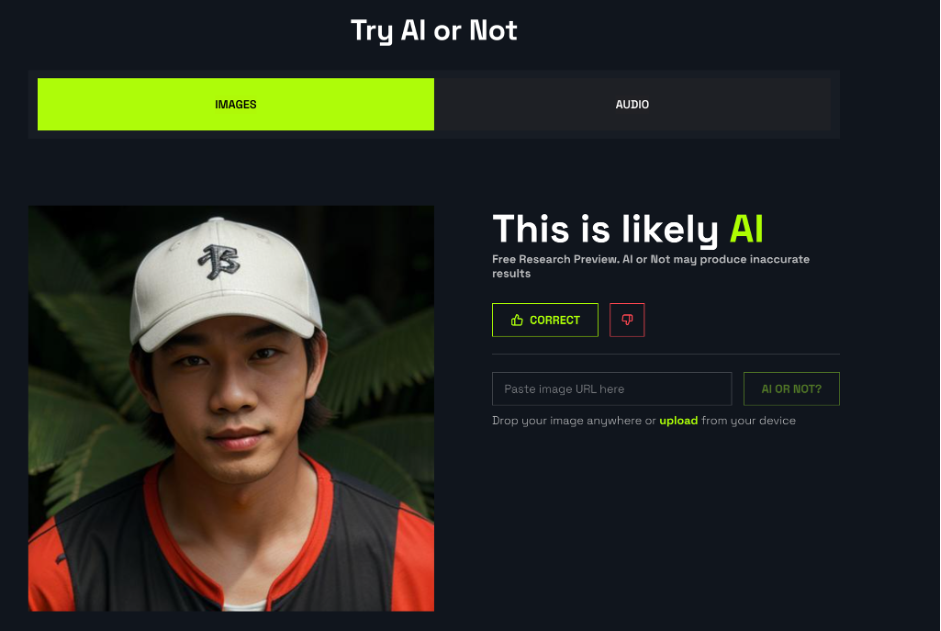
4. Combining Multi-Frame Liveness Detection
- Explanation: This approach leverages the authenticity found in sequential imagery, which is challenging to replicate artificially. Users are prompted to upload a series of images or a short burst, akin to a camera's burst mode or the default Live Photo mode in iPhone cameras. Analyzing these sequences allows for the detection of genuine human movements.
- Example: When a user uploads a Live Photo, and the system's multi-frame analysis discerns authentic human motion, the photo is likely real.
- Con: The effectiveness of this method is contingent upon the type of upload, such as Live Photos or short videos, and may not be as applicable to standard, single-image uploads. However, integrating features like "automatically choose the best shot" can make these multi-frame uploads more appealing and user-friendly.
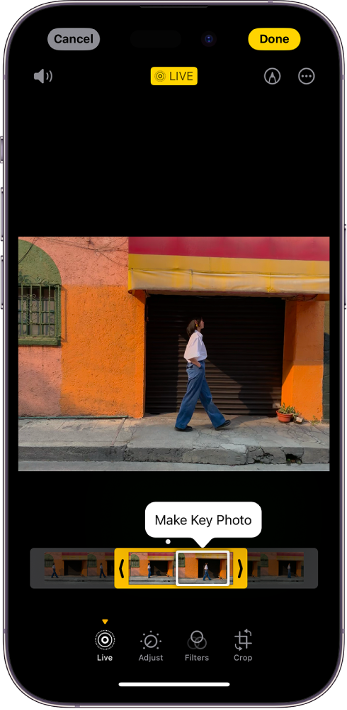
Conclusion
As AI technology evolves, the challenge of distinguishing AI-generated images from real ones grows. While no method is foolproof, a combination of user honesty, data analysis, and advanced AI detection offers a multi-faceted approach to tackling this issue. It's a dynamic field, and continuous advancements are needed to stay ahead of the curve. Which of these methods do you find most effective? Let us know your thoughts!
References:
- Everypixel Journal: https://journal.everypixel.com/ai-image-statistics
- AI or Not: https://www.aiornot.com/
- DALLE2: https://openai.com/dall-e-2
- Apple: https://support.apple.com/en-us/HT207310